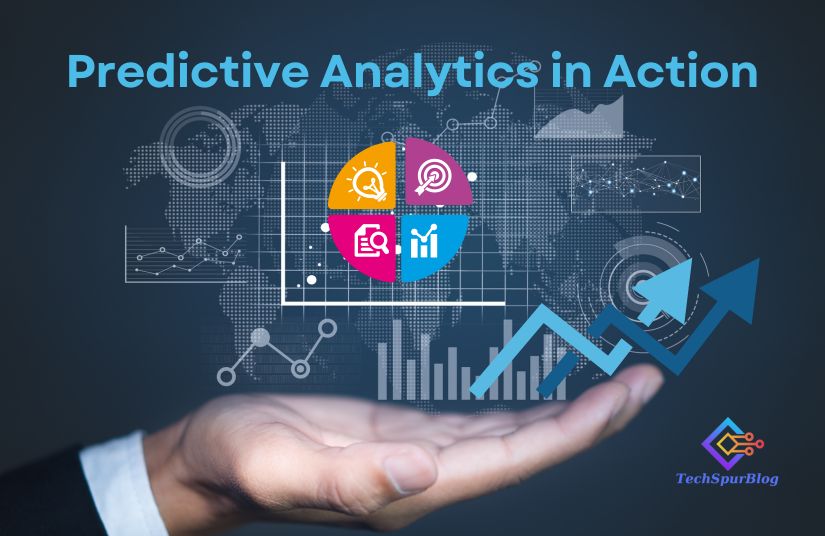
Predicting the future seems like it should be a questionable art limited to astrologers and psychics. However, you don’t have to be psychic to reasonably predict what’s likely to happen if you have reliable data. Think about what meteorologists do. They forecast the weather based on information, tools, and analytics. Some of this data is evolving, while other portions are historical.
This is the foundation of predictive analytics businesses using past data to predict future outcomes. If you’ve never seen it in action, you might wonder how companies accurately pull off what seems questionable at best. This article explores how predictive analytics can forecast what will happen, plus outlines typical and emerging business uses.
Enhancing Marketing Efforts With Better Segmentation
Marketers have long relied on data to segment their audiences. You probably know about demographics and lifestyle factors. Marketers typically use these characteristics to create buyer personas and decide who to target. They determine the audiences likely to respond favorably to specific products or messages and become customers.
Although segmentation has proved beneficial for decades, it can’t always accurately predict an audience’s future behaviors. Say you fit the profile of a company’s target persona. You’re 32 years old, have a college education, and earn over $65k annually. You recently bought your first home and enjoy international travel. But the emails you’ve been getting from cruise lines with international destinations aren’t resonating with you.
A company using predictive audiences would quickly figure this out and why. Maybe you enjoy visiting other countries, but the idea of being on a boat makes you wary. Or, it’s not the right time because you’ve recently started a family. Segmentation using predictive audiences would move you to a do-not-send list until the company strengthens your relationship. In the meantime, the cruise line can focus its efforts on audience segments more likely to convert.
Also Read: How BI and Data Visualization Can Help Your Business
Ensuring Head Count Is Enough
Trying to succeed in a job where you’re overworked is like attempting to push a large boulder up a hill. Eventually, you’ll conclude you should cut your losses. Having too many tasks to accomplish is one of the risk factors for burnout. Multiple competing demands also fall into the category of work overload.
Staffing at the right levels is crucial because 95% of HR leaders say burnout is sabotaging retention. While insufficient headcount means employees are juggling more than they should, the opposite scenario isn’t good either. When staff members become underwhelmed, they can become disconnected from the organization. Feeling like your talents are underused leads to a few of the same negative outcomes as burnout. These consequences include stress and anxiety.
Predictive analytics, particularly in industries where demand fluctuations are the norm, ensures companies staff roles adequately. Tourism and hospitality are some of those industries. The less obvious ones are finance and insurance. Building predictive models for a claims department based on past volume for different states and claim types can help. Additional factors to consider are complexity, average lifecycle, and historical retention rate.
Prevent Infrastructure Failures
When utilities go out for a few minutes, it’s an inconvenience. Increase the timeline to more than four hours and damages to personal property starts to happen. Food can spoil and pipes in a building may freeze. Plus, people’s health may be at risk without heat and air conditioning during extreme temperatures. Businesses people rely on in a pinch, such as convenience stores, may need to close, further impacting health and safety.
Infrastructure failures are a contributing factor to unexpected power outages. Another factor is when demand exceeds supply. Predictive analytics addresses both. Models forecast when equipment is likely to fail so utility companies can be proactive. If you know a transformer is on its last leg, better to address it now than when it goes out.
Past data, such as demand for utilities during cold snaps and heat waves, predicts likely surges. Combining this information with weather forecasts, population changes, and buildings provides a reliable forecast for needed supply. Yet another area where predictive analytics comes into play for utility companies is ensuring worker safety. Models forecast the best working conditions for resource extraction and equipment maintenance.
Also Read: Understanding Business Intelligence: A Detailed Guide For Startups
Increase Road Safety
Vehicle manufacturers and dealerships use predictive analytics to determine maintenance trends. Companies can narrow these trends down to make, model, year, and trim level. With past data, businesses have the insights to identify what preventative maintenance helps drivers avoid trouble down the road.
Most car owners know getting regular oil changes is a must. But what if they knew their car had a 50% chance of breaking down without periodic spark plug changes? These owners would be more motivated to perform this maintenance. Dealerships can offer additional incentives and possibly partner with insurance companies for more savings.
Preventing a breakdown increases safety for the driver plus other citizens using public roadways. Prevention saves insurance companies and vehicle owners money in claims, which add up if injuries or fatalities are involved. Predictive analytics already plays a role in insurance rates but has the potential to expand the scope of risk management.
Predicting the Future With the Past
The past can be a good indicator of what will happen in the future. First, previous behavioral patterns tend to stick. Those patterns typically lead to predictable outcomes. Second, historical information forecasts the probability of identical results occurring again.
Predictive analytics consists of tools companies utilize to improve strategies, relationships with employees, and customer experiences. If your company is behind the learning curve, it’s not too late to catch up. Predicting the future with past data doesn’t take otherworldly abilities. It just involves creative and critical thinking.
Leave a Reply